Overcoming Obstacles: Addressing Common Challenges in AI Implementation
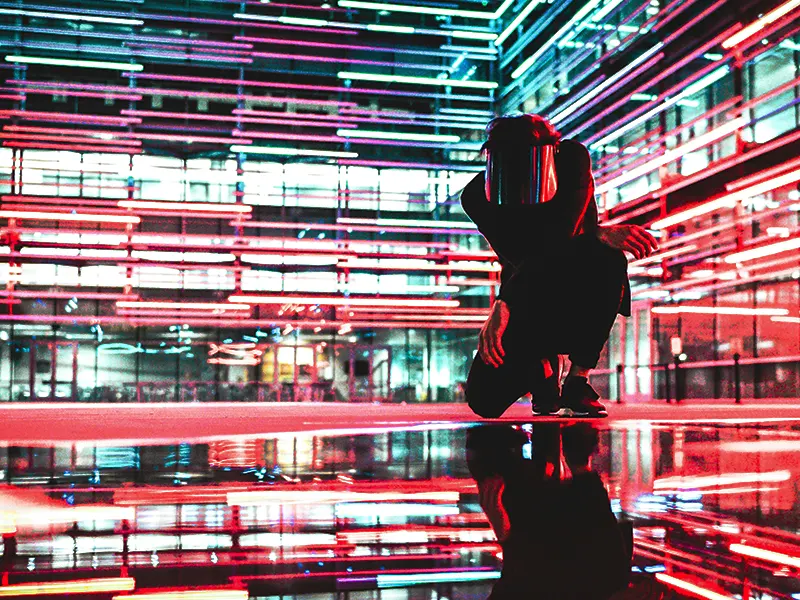
Artificial intelligence (AI) has the potential to revolutionize how businesses operate. From streamlining workflows to powering predictive analytics, AI promises greater efficiency, improved decision-making, and increased profitability. However, implementing AI successfully isn’t a plug-and-play process. For managers in large companies eager to harness the power of AI, it’s critical to understand and proactively tackle some of the most common challenges you’ll likely encounter.
Challenge #1: The Cost Factor
AI development and implementation can be expensive. Businesses need to factor in budgeting for hardware, software, data acquisition, and hiring or upskilling specialized AI personnel. While AI’s ability to reduce costs and boost revenue in the long run is significant, overcoming the initial investment hurdle can be daunting.
Addressing the Cost Challenge:
Start small: Don’t feel pressured to deploy all-encompassing AI solutions at once. Identify a single, well-defined problem that AI can solve efficiently, and focus on demonstrating clear returns.
Consider cloud-based AI: Many cloud providers offer scalable, on-demand AI tools and infrastructure, reducing upfront capital expenditures.
Proof of Concept (POC): Launch a limited pilot project to test an AI solution before fully committing to large-scale deployment. A successful POC helps secure executive buy-in and validates the investment.
Challenge #2: Data Woes
AI systems are fueled by massive amounts of data. Gathering, cleaning, and preparing high-quality datasets can be a time-consuming and resource-intensive process. Additionally, incomplete or biased data can lead to inaccurate AI models, undermining the entire project.
Addressing the Data Challenge:
Data Strategy: Establish a comprehensive plan for data collection, storage, and management early in the AI project. Focus on data quality, not just quantity.
Data Labeling: For supervised learning approaches, ensure your data is accurately labeled. Consider outsourcing this if internal resources are limited.
Bias Mitigation: Actively counter biased data. Assess the diversity and representativeness of datasets to ensure your AI model is accurate and fair.
Challenge #3: Integration Headaches
Integrating AI seamlessly into existing business systems and workflows can be tricky. Compatibility issues, legacy systems, and data silos can complicate the process. A lack of proper integration can limit AI’s effectiveness and cause disruptions if not handled with care.
Addressing the Integration Challenge:
Thorough Assessment: Before implementation, critically evaluate your current infrastructure. Identify potential bottlenecks and compatibility issues to proactively address them.
Phased Implementation: Gradual integration can minimize disruptions, allowing you to test and learn in phases.
APIs and Open Standards: Choose AI solutions that prioritize interoperability, using APIs and open standards to facilitate communication with your existing systems.
Challenge #4: The Talent Gap
Building and managing AI projects requires specialized skills. Finding and attracting top-tier data scientists, AI engineers, and machine learning experts can be challenging, considering the high demand in the market.
Addressing the Talent Challenge:
Upskilling and Reskilling: Invest in training programs for your existing workforce to develop basic AI competencies. Explore online courses and micro-certifications.
Partnerships: Collaborate with external consulting firms or academic institutions to tap into specialized expertise as needed.
Build an Attractive Workplace: To attract top AI talent, cultivate a culture that champions innovation, offers competitive compensation, and provides opportunities for continuous learning.
Challenge #5: Trust and Transparency
Many AI models, particularly deep learning techniques, function as “black boxes.” It can be challenging to understand how they arrive at decisions, impacting trust in the technology. AI’s lack of transparency presents a barrier for managers, especially in regulated industries where explainability is essential.
Addressing the Trust and Transparency Challenge:
Explainable AI (XAI): Explore techniques and tools that aim to make AI models more interpretable. This helps build trust and facilitates compliance.
Monitoring and Auditing: Implement regular reviews and auditing of your AI systems to detect and mitigate bias or unexpected behaviors.
Ethical Frameworks: Establish clear ethical principles throughout your AI development process to guide decision-making and promote responsible AI use.
Conclusion
Successfully implementing AI requires a strategic approach and a willingness to confront these common challenges head-on. By identifying potential obstacles early, addressing them systematically, and cultivating a culture of data-driven innovation, managers in large companies can position their organizations to fully reap the benefits of AI and drive sustainable competitive advantage in the years to come.